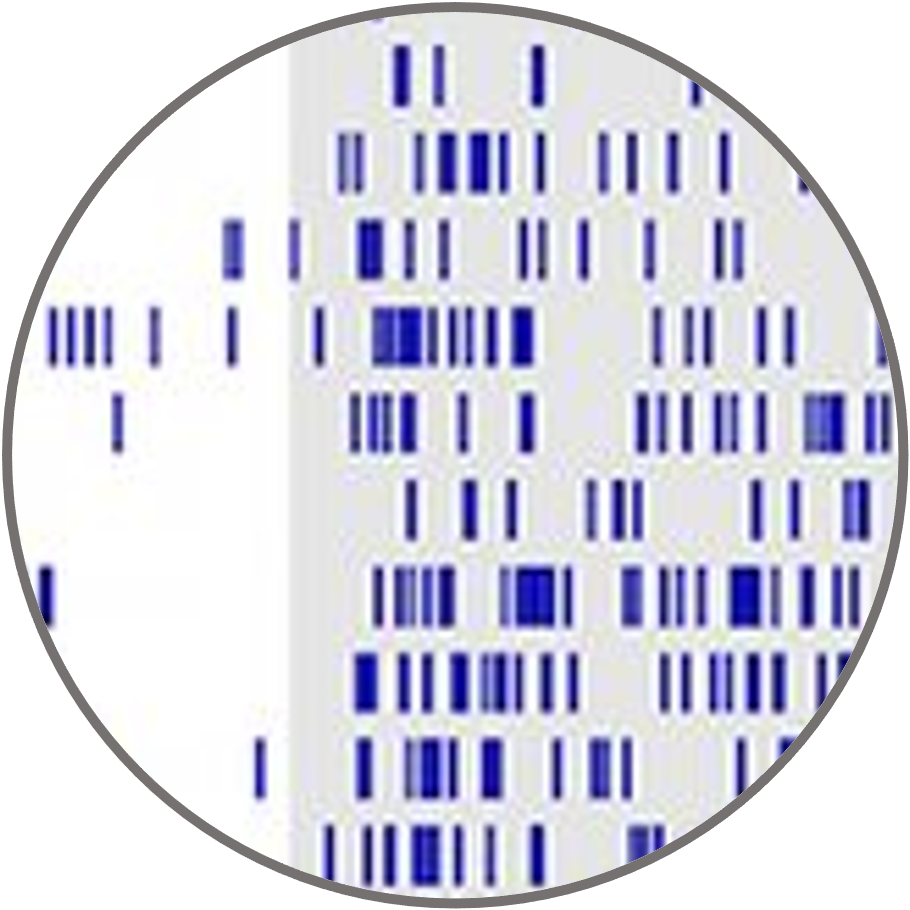
Sensory Processing & Energy Homeostasis
Olfaction is a major research topic in sensory neuroscience. We study the neural computation underlying olfactory processing and multisensory integration in the insect brain combining experimental and computational modeling approaches. In biologically realistic neural network models we achieve the transformation from peripheral dense coding to efficient and multimodal sparse codes. Sparse coding is specifically important for the formation and retrieval of associative memories. Our models are based on and/or validated through experimental data.
Publications
-
Sakagiannis, P. P., Rapp, H., Jovanic, T., & Nawrot, M. P.. (2025). Larvaworld : A behavioral simulation and analysis platform for Drosophila larva. bioRxiv, 2025.06.15.659765.
[Bibtex]@article {Sakagiannis2025.06.15.659765, author = {Sakagiannis, Panagiotis Parthenios and Rapp, Hannes and Jovanic, Tihana and Nawrot, Martin Paul}, title = {Larvaworld : A behavioral simulation and analysis platform for Drosophila larva}, elocation-id = {2025.06.15.659765}, year = {2025}, doi = {10.1101/2025.06.15.659765}, publisher = {Cold Spring Harbor Laboratory}, abstract = {Behavioral modeling supports theory building and evaluation across disciplines. Leveraging advances in motion-tracking and computational tools, we present a virtual laboratory for Drosophila larvae that integrates agent-based modeling with multiscale neural control and supports analysis of both simulated and experimental data. Virtual larvae are implemented as 2D agents capable of realistic locomotion, guided by multimodal sensory input and constrained by a dynamic energy-budget model that balances exploration and exploitation. Each agent is organized as a hierarchical, behavior-based control system comprising three layers: low-level locomotion, optionally incorporating neuromechanical models; mid-level sensory processing; and high-level behavioral adaptation. Neural control models can range from simple linear transfer models to rate-based or spiking neural network models, e.g. to accomodate associative learning. Simulations operate across sub-millisecond neuronal dynamics, sub-second closed-loop behavior, and circadian-scale metabolic regulation. Users can configure both larval models and virtual environments, including sensory landscapes, nutrient sources, and physical arenas. Real-time visualization is integrated into the simulation and analysis pipeline, which also allows for standardized processing of motion-tracking data from real experiments. Distributed as an open-source Python package, the platform includes tutorial experiments to support accessibility, customization, and use in both research and education.Competing Interest StatementThe authors have declared no competing interest.German Federal Ministry of Education and Research (BMBF), 01GQ2103AFrench National Research Agency (ANR), ANR-21-NEUC-0002United States Department of Energy (DOE), SC0021922French National Research Agency (ANR), ANR-22-CE37-0027F{\'e}d{\'e}ration pour la Recherche sur le Cerveau, https://ror.org/005zda847Fondation pour la Recherche M{\'e}dicale (FRM), EQU202303016317Ministry of Culture and Science of the State of Northrhine Westphalia, Netzwerke 2021 (iBehave)}, URL = {https://www.biorxiv.org/content/early/2025/06/18/2025.06.15.659765}, eprint = {https://www.biorxiv.org/content/early/2025/06/18/2025.06.15.659765.full.pdf}, journal = {bioRxiv}, pages = {2025.06.15.659765} }
-
Sakagiannis, P., Jürgensen, A., & Nawrot, M. P.. (2025). A behavioral architecture for realistic simulations of Drosophila larva locomotion and foraging. eLife, 14, RP104262.
[Bibtex]@article{Sakagiannis_2025, title={A behavioral architecture for realistic simulations of Drosophila larva locomotion and foraging}, url={http://dx.doi.org/10.7554/eLife.104262.1}, DOI={10.7554/elife.104262.1}, publisher={eLife Sciences Publications, Ltd}, author={Sakagiannis, Panagiotis and Jürgensen, Anna-Maria and Nawrot, Martin Paul}, year={2025}, journal = {eLife}, volume = {14}, pages = {RP104262},month=apr }
-
Tredern, E. D., Manceau, D., Blanc, A., Sakagiannis, P., Barre, C., Sus, V., Viscido, F., Hasa, M. H., Autran, S., Nawrot, M. P., Masson, J., & Jovanic, T.. (2023). Feeding- state dependent modulation of reciprocally interconnected inhibitory neurons biases sensorimotor decisions in Drosophila. bioRxiv.
[Bibtex]@article{Tredern2023, author = {Tredern, Elo{\"{i}}se De and Manceau, Dylan and Blanc, Alexandre and Sakagiannis, Panagiotis and Barre, Chloe and Sus, Victoria and Viscido, Francesca and Hasa, Md Hamit and Autran, Sandra and Nawrot, Martin Paul and Masson, Jean-Baptiste and Jovanic, Tihana}, doi = {10.1101/2023.12.26.573306}, file = {:Users/springer/Downloads/2023.12.26.573306v1.full.pdf:pdf}, journal = {bioRxiv}, month = {dec}, title = {{Feeding- state dependent modulation of reciprocally interconnected inhibitory neurons biases sensorimotor decisions in Drosophila}}, url = {https://doi.org/10.1101/2023.12.26.573306}, year = {2023} }
-
Arican, C., Schmitt, F. J., Rössler, W., Strube-Bloss, M. F., & Nawrot, M. P.. (2023). The mushroom body output encodes behavioral decision during sensory-motor transformation. Current Biology, 33, 1–8.
[Bibtex]@article{Arican2022, abstract = {Animal behavioral decisions are dynamically formed by evaluating momentary sensory evidence on the background of individual experience and the acute motivational state. In insects, the mushroom body (MB) has been implicated in forming associative memories and in assessing the appetitive or aversive valence of sensory stimuli to bias approach versus avoidance behavior. To study the MB involvement in innate feeding behavior we performed extracellular single-unit recordings from MB output neurons (MBONs) while simultaneously monitoring a defined feeding behavior in response to timed odor stimulation in naive cockroaches. All animals expressed the feeding behavior almost exclusively in response to food odors. Likewise, MBON responses were invariably and strongly tuned to the same odors. Importantly, MBON responses were restricted to behaviorally responded trials, which allowed the accurate prediction of the occurrence versus non-occurrence of the feeding behavior in individual trials from the neuronal population activity. During responded trials the neuronal activity generally preceded the onset of the feeding behavior, indicating a causal relation. Our results contest the predominant view that MBONs encode stimulus valence. Rather, we conclude that the MB output dynamically encodes the behavioral decision to inform downstream motor networks.Competing Interest StatementThe authors have declared no competing interest.}, author = {Arican, Cansu and Schmitt, Felix Johannes and R{\"{o}}ssler, Wolfgang and Strube-Bloss, Martin Fritz and Nawrot, Martin Paul}, doi = {10.1016/j.cub.2023.08.016}, file = {:Users/springer/Downloads/PIIS096098222301059X.pdf:pdf}, journal = {Current Biology}, month = {sep}, pages = {1--8}, title = {{The mushroom body output encodes behavioral decision during sensory-motor transformation}}, url = {https://www.cell.com/current-biology/fulltext/S0960-9822(23)01059-X}, volume = {33}, year = {2023} }
-
Sakagiannis, P., Aguilera, M., & Nawrot, M. P.. (2020). A Plausible Mechanism for Drosophila Larva Intermittent Behavior. In Vouloutsi, V., Mura, A., Tauber, F., Speck, T., Prescott, T. J., & Verschure, P. F. M. J. (Eds.), In Biomimetic and Biohybrid Systems. Living Machines 2020. Lecture Notes in Computer Science .
[Bibtex]@incollection{Sakagiannis2020, abstract = {The behavior of many living organisms is not continuous. Rather, activity emerges in bouts that are separated by epochs of rest, a phenomenon known as intermittent behavior. Although intermit- tency is ubiquitous across phyla, empirical studies are scarce and the underlying neural mechanisms remain unknown. Here we re- produce empirical evidence of intermittency during Drosophila larva free exploration. Our findings are in line with previously reported power-law distributed rest-bout durations while we report log-normal distributed activity-bout durations. We show that a stochastic net- work model can transition between power-law and non-power-law distributed states and we suggest a plausible neural mechanism for the alternating rest and activity in the larva. Finally, we discuss pos- sible implementations in behavioral simulations extending spatial Levy-walk or coupled-oscillator models with temporal intermittency}, author = {Sakagiannis, Panagiotis and Aguilera, Miguel and Nawrot, Martin Paul}, booktitle = {Biomimetic and Biohybrid Systems. Living Machines 2020. Lecture Notes in Computer Science}, doi = {10.1007/978-3-030-64313-3_28}, editor = {Vouloutsi, V. and Mura, A. and Tauber, F. and Speck, T. and Prescott, T.J. and Verschure, P.F.M.J.}, file = {:Users/springer/Library/Application Support/Mendeley Desktop/Downloaded/Sakagiannis, Aguilera, Nawrot - 2020 - A Plausible Mechanism for Drosophila Larva Intermittent Behavior(2).pdf:pdf}, keywords = {larva crawling,levy-walks,neuronal avalanches}, month = {dec}, title = {{A Plausible Mechanism for Drosophila Larva Intermittent Behavior}}, url = {https://doi.org/10.1007/978-3-030-64313-3{\_}28}, year = {2020} }
-
Rapp, H., Nawrot, M. P., & Stern, M.. (2020). Numerical Cognition Based on Precise Counting with a Single Spiking Neuron. iScience, 23(2), 100852.
[Bibtex]@article{Rapp2020, abstract = {Insects are able to solve basic numerical cognition tasks. We show that estimation of numerosity can be realized and learned by a single spiking neuron with an appropriate synaptic plasticity rule. This model can be efficiently trained to detect arbitrary spatiotemporal spike patterns on a noisy and dynamic background with high precision and low variance. When put to test in a task that requires counting of visual concepts in a static image it required considerably less training epochs than a convolutional neural network to achieve equal performance. When mimicking a behavioral task in free-flying bees that requires numerical cognition, the model reaches a similar success rate in making correct decisions. We propose that using action potentials to represent basic numerical concepts with a single spiking neuron is beneficial for organisms with small brains and limited neuronal resources.}, author = {Rapp, Hannes and Nawrot, Martin Paul and Stern, Merav}, doi = {10.1016/j.isci.2020.100852}, file = {:Users/springer/Library/Application Support/Mendeley Desktop/Downloaded/Rapp, Nawrot, Stern - 2020 - Numerical Cognition Based on Precise Counting with a Single Spiking Neuron.pdf:pdf}, issn = {25890042}, journal = {iScience}, keywords = {Cognitive Neuroscience,In Silico Biology,Neuroscience}, month = {feb}, number = {2}, pages = {100852}, publisher = {Elsevier Inc.}, title = {{Numerical Cognition Based on Precise Counting with a Single Spiking Neuron}}, url = {https://doi.org/10.1016/j.isci.2020.100852}, volume = {23}, year = {2020} }
-
Nawrot, M. P., Kloppenburg, P., & Deger, M.. (2018). Foreword for the special issue on Neural Coding. Biological Cybernetics, 112(1-2), 11.
[Bibtex]@article{Nawrot2018, author = {Nawrot, Martin P. and Kloppenburg, Peter and Deger, Moritz}, doi = {10.1007/s00422-018-0754-1}, file = {::}, issn = {14320770}, journal = {Biological Cybernetics}, number = {1-2}, pages = {11}, title = {{Foreword for the special issue on Neural Coding}}, volume = {112}, year = {2018} }
-
Kumar, R., Kaur, R., Auffarth, B., & Bhondekar, A. P.. (2015). Understanding the odour spaces: A step towards solving olfactory stimulus-percept problem. PLoS ONE, 10(10), 1–19.
[Bibtex]@article{Kumar2015, abstract = {Odours are highly complex, relying on hundreds of receptors, and people are known to disagree in their linguistic descriptions of smells. It is partly due to these facts that, it is very hard to map the domain of odour molecules or their structure to that of perceptual representations, a problem that has been referred to as the Structure-Odour-Relationship. We collected a number of diverse open domain databases of odour molecules having unorganised perceptual descriptors, and developed a graphical method to find the similarity between perceptual descriptors; which is intuitive and can be used to identify perceptual classes. We then separately projected the physico-chemical and perceptual features of these molecules in a non-linear dimension and clustered the similar molecules.We found a significant overlap between the spatial positioning of the clustered molecules in the physico-chemical and perceptual spaces.We also developed a statistical method of predicting the perceptual qualities of a novel molecule using its physico-chemical properties with high receiver operating characteristics(ROC).}, author = {Kumar, Ritesh and Kaur, Rishemjit and Auffarth, Benjamin and Bhondekar, Amol P.}, doi = {10.1371/journal.pone.0141263}, file = {:Users/springer/Desktop/journal.pone.0141263.pdf:pdf}, issn = {19326203}, journal = {PLoS ONE}, number = {10}, pages = {1--19}, title = {{Understanding the odour spaces: A step towards solving olfactory stimulus-percept problem}}, volume = {10}, year = {2015} }
-
Kloppenburg, P., & Nawrot, M. P.. (2014). Neural coding: Sparse but on time. Current Biology, 24(19), R957–R959.
[Bibtex]@article{Kloppenburg2014, abstract = {To code information efficiently, sensory systems use sparse representations. In a sparse code, a specific stimulus activates only few spikes in a small number of neurons. A new study shows that the temporal pattern across sparsely activated neurons encodes information, suggesting that the sparse code extends into the time domain.}, author = {Kloppenburg, Peter and Nawrot, Martin Paul}, doi = {10.1016/j.cub.2014.08.041}, file = {:Users/springer/Library/Application Support/Mendeley Desktop/Downloaded/Kloppenburg, Nawrot - 2014 - Neural coding Sparse but on time.pdf:pdf}, issn = {09609822}, journal = {Current Biology}, keywords = {insect olfaction,rate model,sparse coding}, mendeley-tags = {insect olfaction,rate model,sparse coding}, number = {19}, pages = {R957--R959}, title = {{Neural coding: Sparse but on time}}, volume = {24}, year = {2014} }
-
Meckenhäuser, G., Krämer, S., Farkhooi, F., Ronacher, B., & Nawrot, M. P.. (2014). Neural representation of calling songs and their behavioral relevance in the grasshopper auditory system. Frontiers in Systems Neuroscience, 8, 183.
[Bibtex]@article{Meckenhauser2014, abstract = {{\textcopyright} 2014 Meckenh{\"{a}}user, Kr{\"{a}}mer, Farkhooi, Ronacher and Nawrot. Acoustic communication plays a key role for mate attraction in grasshoppers. Males use songs to advertise themselves to females. Females evaluate the song pattern, a repetitive structure of sound syllables separated by short pauses, to recognize a conspecific male and as proxy to its fitness. In their natural habitat females often receive songs with degraded temporal structure. Perturbations may, for example, result from the overlap with other songs. We studied the response behavior of females to songs that show different signal degradations. A perturbation of an otherwise attractive song at later positions in the syllable diminished the behavioral response, whereas the same perturbation at the onset of a syllable did not affect song attractiveness. We applied na{\"{i}}ve Bayes classifiers to the spike trains of identified neurons in the auditory pathway to explore how sensory evidence about the acoustic stimulus and its attractiveness is represented in the neuronal responses. We find that populations of three or more neurons were sufficient to reliably decode the acoustic stimulus and to predict its behavioral relevance from the single-trial integrated firing rate. A simple model of decision making simulates the female response behavior. It computes for each syllable the likelihood for the presence of an attractive song pattern as evidenced by the population firing rate. Integration across syllables allows the likelihood to reach a decision threshold and to elicit the behavioral response. The close match between model performance and animal behavior shows that a spike rate code is sufficient to enable song pattern recognition.}, author = {Meckenh{\"{a}}user, Gundula and Kr{\"{a}}mer, Stefanie and Farkhooi, Farzad and Ronacher, Bernhard and Nawrot, Martin P.}, doi = {10.3389/fnsys.2014.00183}, file = {::}, issn = {16625137}, journal = {Frontiers in Systems Neuroscience}, keywords = {Acoustic communication,Decision making,Na{\"{i}}ve Bayes classifier,Neural information processing,Pattern recognition,Population coding}, pages = {183}, title = {{Neural representation of calling songs and their behavioral relevance in the grasshopper auditory system}}, volume = {8}, year = {2014} }
-
Farkhooi, F., Froese, A., Muller, E., Menzel, R., & Nawrot, M. P.. (2013). Cellular Adaptation Facilitates Sparse and Reliable Coding in Sensory Pathways. PLoS Computational Biology, 9(10), e1003251.
[Bibtex]@article{Farkhooi2013, abstract = {Most neurons in peripheral sensory pathways initially respond vigorously when a preferred stimulus is presented, but adapt as stimulation continues. It is unclear how this phenomenon affects stimulus coding in the later stages of sensory processing. Here, we show that a temporally sparse and reliable stimulus representation develops naturally in sequential stages of a sensory network with adapting neurons. As a modeling framework we employ a mean-field approach together with an adaptive population density treatment, accompanied by numerical simulations of spiking neural networks. We find that cellular adaptation plays a critical role in the dynamic reduction of the trial-by-trial variability of cortical spike responses by transiently suppressing self-generated fast fluctuations in the cortical balanced network. This provides an explanation for a widespread cortical phenomenon by a simple mechanism. We further show that in the insect olfactory system cellular adaptation is sufficient to explain the emergence of the temporally sparse and reliable stimulus representation in the mushroom body. Our results reveal a generic, biophysically plausible mechanism that can explain the emergence of a temporally sparse and reliable stimulus representation within a sequential processing architecture.}, author = {Farkhooi, Farzad and Froese, Anja and Muller, Eilif and Menzel, Randolf and Nawrot, Martin P.}, doi = {10.1371/journal.pcbi.1003251}, file = {:Users/springer/Library/Application Support/Mendeley Desktop/Downloaded/Farkhooi et al. - 2013 - Cellular Adaptation Facilitates Sparse and Reliable Coding in Sensory Pathways.pdf:pdf}, issn = {1553734X}, journal = {PLoS Computational Biology}, number = {10}, pages = {e1003251}, title = {{Cellular Adaptation Facilitates Sparse and Reliable Coding in Sensory Pathways}}, volume = {9}, year = {2013} }
-
Meckenhäuser, G., Hennig, M. R., & Nawrot, M. P.. (2013). Critical Song Features for Auditory Pattern Recognition in Crickets. PLoS ONE, 8(2).
[Bibtex]@article{Meckenhauser2013, abstract = {Many different invertebrate and vertebrate species use acoustic communication for pair formation. In the cricket Gryllus bimaculatus, females recognize their species-specific calling song and localize singing males by positive phonotaxis. The song pattern of males has a clear structure consisting of brief and regular pulses that are grouped into repetitive chirps. Information is thus present on a short and a long time scale. Here, we ask which structural features of the song critically determine the phonotactic performance. To this end we employed artificial neural networks to analyze a large body of behavioral data that measured females' phonotactic behavior under systematic variation of artificially generated song patterns. In a first step we used four non-redundant descriptive temporal features to predict the female response. The model prediction showed a high correlation with the experimental results. We used this behavioral model to explore the integration of the two different time scales. Our result suggested that only an attractive pulse structure in combination with an attractive chirp structure reliably induced phonotactic behavior to signals. In a further step we investigated all feature sets, each one consisting of a different combination of eight proposed temporal features. We identified feature sets of size two, three, and four that achieve highest prediction power by using the pulse period from the short time scale plus additional information from the long time scale. {\textcopyright} 2013 Meckenh{\"{a}}user et al.}, author = {Meckenh{\"{a}}user, Gundula and Hennig, R. Matthias and Nawrot, Martin P.}, doi = {10.1371/journal.pone.0055349}, file = {::}, issn = {19326203}, journal = {PLoS ONE}, number = {2}, title = {{Critical Song Features for Auditory Pattern Recognition in Crickets}}, volume = {8}, year = {2013} }
-
Häusler, C., Susemihl, A., & Nawrot, M. P.. (2013). Natural image sequences constrain dynamic receptive fields and imply a sparse code. Brain Research, 1536, 53–67.
[Bibtex]@article{Hausler2013, abstract = {In their natural environment, animals experience a complex and dynamic visual scenery. Under such natural stimulus conditions, neurons in the visual cortex employ a spatially and temporally sparse code. For the input scenario of natural still images, previous work demonstrated that unsupervised feature learning combined with the constraint of sparse coding can predict physiologically measured receptive fields of simple cells in the primary visual cortex. This convincingly indicated that the mammalian visual system is adapted to the natural spatial input statistics. Here, we extend this approach to the time domain in order to predict dynamic receptive fields that can account for both spatial and temporal sparse activation in biological neurons. We rely on temporal restricted Boltzmann machines and suggest a novel temporal autoencoding training procedure. When tested on a dynamic multi-variate benchmark dataset this method outperformed existing models of this class. Learning features on a large dataset of natural movies allowed us to model spatio-temporal receptive fields for single neurons. They resemble temporally smooth transformations of previously obtained static receptive fields and are thus consistent with existing theories. A neuronal spike response model demonstrates how the dynamic receptive field facilitates temporal and population sparseness. We discuss the potential mechanisms and benefits of a spatially and temporally sparse representation of natural visual input. {\textcopyright} 2013 The Authors.}, author = {H{\"{a}}usler, Chris and Susemihl, Alex and Nawrot, Martin P.}, doi = {10.1016/j.brainres.2013.07.056}, file = {::}, issn = {00068993}, journal = {Brain Research}, keywords = {Autoencoding,Lifetime sparseness,Machine learning,Population sparseness,Restricted Boltzmann Machine,Visual cortex}, pages = {53--67}, title = {{Natural image sequences constrain dynamic receptive fields and imply a sparse code}}, volume = {1536}, year = {2013} }
-
Brill, M. F., Rosenbaum, T., Reus, I., Kleineidam, C. J., Nawrot, M. P., & Rössler, W.. (2013). Parallel processing via a dual olfactory pathway in the honeybee. Journal of Neuroscience, 33(6), 2443–2456.
[Bibtex]@article{Brill2013, abstract = {In their natural environment, animals face complex and highly dynamic olfactory input. Thus vertebrates as well as invertebrates require fast and reliable processing of olfactory information. Parallel processing has been shown to improve processing speed and power in other sensory systems and is characterized by extraction of different stimulus parameters along parallel sensory information streams. Honeybees possess an elaborate olfactory system with unique neuronal architecture: a dual olfactory pathway comprising a medial projection-neuron (PN) antennal lobe (AL) protocerebral output tract (m-APT) and a lateral PN AL output tract (l-APT) connecting the olfactory lobes with higher-order brain centers. We asked whether this neuronal architecture serves parallel processing and employed a novel technique for simultaneous multiunit recordings from both tracts. The results revealed response profiles from a high number of PNs of both tracts to floral, pheromonal, and biologically relevant odor mixtures tested over multiple trials. PNs from both tracts responded to all tested odors, but with different characteristics indicating parallel processing of similar odors. Both PN tracts were activated by widely overlapping response profiles, which is a requirement for parallel processing. The l-APT PNs had broad response profiles suggesting generalized coding properties, whereas the responses of m-APT PNs were comparatively weaker and less frequent, indicating higher odor specificity. Comparison of response latencies within and across tracts revealed odor-dependent latencies. We suggest that parallel processing via the honeybee dual olfactory pathway provides enhanced odor processing capabilities serving sophisticated odor perception and olfactory demands associated with a complex olfactory world of this social insect.}, author = {Brill, Martin F. and Rosenbaum, Tobias and Reus, Isabelle and Kleineidam, Christoph J. and Nawrot, Martin P. and R{\"{o}}ssler, Wolfgang}, doi = {10.1523/JNEUROSCI.4268-12.2013}, file = {:Users/springer/Documents/PhD/Lit/Brill (2013) Parallel Processing via a Dual Olfactory Pathway in the Honeybee.pdf:pdf}, issn = {02706474}, journal = {Journal of Neuroscience}, keywords = {insect olfaction,review paper}, mendeley-tags = {insect olfaction,review paper}, number = {6}, pages = {2443--2456}, title = {{Parallel processing via a dual olfactory pathway in the honeybee}}, volume = {33}, year = {2013} }
-
Berger, D., Pazienti, A., Flores, F. J., Nawrot, M. P., Maldonado, P. E., & Grün, S.. (2012). Viewing strategy of Cebus monkeys during free exploration of natural images. Brain Research, 1434, 34–46.
[Bibtex]@article{Berger2012, abstract = {Humans and other primates move their eyes several times per second to foveate at different locations of a visual scene. What features of a scene guide eye movements in natural vision? We recorded eye movements of three monkeys during free exploration of natural scenes and propose a simple model to explain their dynamics. We use the spatial clustering of fixation positions to define the monkeys' subjective regions-of-interest (ROI) in natural scenes. For most images the subjective ROIs match significantly the computed saliency of the natural scene, except when the image contains human or primate faces. We also investigated the temporal sequence of eye movements by computing the probability that a fixation will be made inside or outside of the ROI, given the current fixation position. We fitted a Markov chain model to the sequence of fixation positions, and find that fixations made inside a ROI are more likely to be followed by another fixation in the same ROI. This is true, independent of the image saliency in the area of the ROI. Our results show that certain regions in a natural scene are explored locally before directing the focus to another local region. This strategy could allow for quick integration of the visual features that constitute an object, and efficient segmentation of objects from other objects and the background during free viewing of natural scenes. {\textcopyright} 2011 Elsevier B.V. All rights reserved.}, author = {Berger, Denise and Pazienti, Antonio and Flores, Francisco J. and Nawrot, Martin P. and Maldonado, Pedro E. and Gr{\"{u}}n, Sonja}, doi = {10.1016/j.brainres.2011.10.013}, file = {::}, issn = {00068993}, journal = {Brain Research}, keywords = {Eye movement,Fixation map,Monkey,Natural vision,Scan path}, pages = {34--46}, title = {{Viewing strategy of Cebus monkeys during free exploration of natural images}}, volume = {1434}, year = {2012} }
- Nawrot, M. P.. (2011). Neuroinformatik: Theorie Neuronaler Informationsverarbeitung.. In Speerspitzen (pp. 184–187). Heidelberger Akademie der Wissenschaften (Hrsg.), Universitätsverlag Winter, Heidelberg.
[Bibtex]@incollection{Nawrot2011, author = {Nawrot, Martin Paul}, booktitle = {Speerspitzen}, pages = {184--187}, publisher = {Heidelberger Akademie der Wissenschaften (Hrsg.), Universit{\"{a}}tsverlag Winter, Heidelberg}, title = {{Neuroinformatik: Theorie Neuronaler Informationsverarbeitung.}}, url = {https://www.bcp.fu-berlin.de/en/biologie/arbeitsgruppen/Archiv/ag{\_}nawrot/research/pdf/Nawrot11{\_}184.pdf}, year = {2011} }
-
Meckenhäuser, G., Hennig, M. R., & Nawrot, M. P.. (2011). Modeling phonotaxis in female Gryllus bimaculatus with artificial neural networks. Paper presented at the BMC Neuroscience.
[Bibtex]@inproceedings{Meckenhauser2011, author = {Meckenh{\"{a}}user, Gundula and Hennig, Matthias R and Nawrot, Martin P}, booktitle = {BMC Neuroscience}, doi = {10.1186/1471-2202-12-s1-p234}, file = {:Users/springer/Downloads/Meckenh{\~{A}}¤user2011{\_}Article{\_}ModelingPhonotaxisInFemaleGryl.pdf:pdf}, issn = {1471-2202}, number = {S1}, pages = {P234}, title = {{Modeling phonotaxis in female Gryllus bimaculatus with artificial neural networks}}, volume = {12}, year = {2011} }
- Nawrot, M. P., Krofczik, S., Farkhooi, F., & Menzel, R.. (2010). Fast dynamics of odor rate coding in the insect antennal lobe. arXiv, 1101.0271.
[Bibtex]@article{Nawrot2010, abstract = {Insects identify and evaluate behaviorally relevant odorants in complex natural scenes where odor concentrations and mixture composition can change rapidly. In the honeybee, a combinatorial code of activated and inactivated projection neurons (PNs) develops rapidly within tens of milliseconds at the first level of neural integration, the antennal lobe (AL). The phasic-tonic stimulus-response dynamics observed in the neural population code and in the firing rate profiles of single neurons is faithfully captured by two alternative models which rely either on short-term synaptic depression, or on spike frequency adaptation. Both mechanisms work independently and possibly in parallel to lateral inhibition. Short response latencies in local interneurons indicate that local processing within the AL network relies on fast lateral inhibition that can suppress effectively and specifically odor responses in single PNs. Reviewing recent findings obtained in different insect species, we conclude that the insect olfactory system implements a fast and reliable coding scheme optimized for time-varying input within the behaviorally relevant dynamic range.}, archivePrefix = {arXiv}, arxivId = {1101.0271}, author = {Nawrot, Martin Paul and Krofczik, Sabine and Farkhooi, Farzad and Menzel, Randolf}, eprint = {1101.0271}, file = {:Users/springer/Downloads/1101.0271.pdf:pdf}, journal = {arXiv}, keywords = {combinatorial code,frequency adaptation,honeybee,latency code,lateral inhibition,olfaction,short term depression,spike}, pages = {1101.0271}, title = {{Fast dynamics of odor rate coding in the insect antennal lobe}}, url = {http://arxiv.org/abs/1101.0271}, year = {2010} }
-
Farkhooi, F., Strube-Bloss, M. F., & Nawrot, M. P.. (2009). Serial correlation in neural spike trains: Experimental evidence, stochastic modeling, and single neuron variability. Physical Review E – Statistical, Nonlinear, and Soft Matter Physics, 79(2), 21905.
[Bibtex]@article{Farkhooi2009, abstract = {The activity of spiking neurons is frequently described by renewal point process models that assume the statistical independence and identical distribution of the intervals between action potentials. However, the assumption of independent intervals must be questioned for many different types of neurons. We review experimental studies that reported the feature of a negative serial correlation of neighboring intervals, commonly observed in neurons in the sensory periphery as well as in central neurons, notably in the mammalian cortex. In our experiments we observed the same short-lived negative serial dependence of intervals in the spontaneous activity of mushroom body extrinsic neurons in the honeybee. To model serial interval correlations of arbitrary lags, we suggest a family of autoregressive point processes. Its marginal interval distribution is described by the generalized gamma model, which includes as special cases the log-normal and gamma distributions, which have been widely used to characterize regular spiking neurons. In numeric simulations we investigated how serial correlation affects the variance of the neural spike count. We show that the experimentally confirmed negative correlation reduces single-neuron variability, as quantified by the Fano factor, by up to 50{\%}, which favors the transmission of a rate code. We argue that the feature of a negative serial correlation is likely to be common to the class of spike-frequency- adapting neurons and that it might have been largely overlooked in extracellular single-unit recordings due to spike sorting errors. {\textcopyright} 2009 The American Physical Society.}, author = {Farkhooi, F. and Strube-Bloss, M.F. and Nawrot, M.P.}, doi = {10.1103/PhysRevE.79.021905}, file = {::}, issn = {15393755}, journal = {Physical Review E - Statistical, Nonlinear, and Soft Matter Physics}, keywords = {Fano factor,spike train statistics,spiking irregularity}, mendeley-tags = {Fano factor,spike train statistics,spiking irregularity}, number = {2}, pages = {021905}, title = {{Serial correlation in neural spike trains: Experimental evidence, stochastic modeling, and single neuron variability}}, volume = {79}, year = {2009} }
-
Krofczik, S., Menzel, R., & Nawrot, M. P.. (2009). Rapid odor processing in the honeybee antennal lobe network. Frontiers in Computational Neuroscience, 2, 9.
[Bibtex]@article{Krofczik2009, abstract = {In their natural environment, many insects need to identify and evaluate behaviorally relevant odorants on a rich and dynamic olfactory background. Behavioral studies have demonstrated that bees recognize learned odors within {\textless}200 ms, indicating a rapid processing of olfactory input in the sensory pathway. We studied the role of the honeybee antennal lobe network in constructing a fast and reliable code of odor identity using in vivo intracellular recordings of individual projection neurons (PNs) and local interneurons (LNs). We found a complementary ensemble code where odor identity is encoded in the spatio-temporal pattern of response latencies as well as in the pattern of activated and inactivated PN firing. This coding scheme rapidly reaches a stable representation within 50-150 ms after stimulus onset. Testing an odor mixture versus its individual compounds revealed different representations in the two morphologically distinct types of lateral- and median PNs (l- and m-PNs). Individual m-PNs mixture responses were dominated by the most effective compound (elemental representation) whereas l-PNs showed suppressed responses to the mixture but not to its individual compounds (synthetic representation). The onset of inhibition in the membrane potential of l-PNs coincided with the responses of putative inhibitory interneurons that responded significantly faster than PNs. Taken together, our results suggest that processing within the LN network of the AL is an essential component of constructing the antennal lobe population code. {\textcopyright} 2009 Krofczik, Menzel and Nawrot.}, author = {Krofczik, S. and Menzel, R. and Nawrot, M.P.}, doi = {10.3389/neuro.10.009.2008}, file = {:Users/springer/Documents/PhD/Lit/Krofczik (2009) Rapid odor processing in the honeybee antennal lobe network.pdf:pdf}, issn = {16625188}, journal = {Frontiers in Computational Neuroscience}, keywords = {Antennal lobe,Apis mellifera,Latency code,Local interneurons,Odor mixture,Olfaction,Projection neurons,Temporal coding}, pages = {9}, title = {{Rapid odor processing in the honeybee antennal lobe network}}, volume = {2}, year = {2009} }
-
Rybak, J., Kuß, A., Holler, W., Brandt, R., Hege, H., Nawrot, M. P., & Menzel, R.. (2009). The HoneyBee Standard Brain (HSB) – a versatile atlas tool for integrating data and data exchange in the neuroscience community. Paper presented at the BMC Neuroscience.
[Bibtex]@inproceedings{Krofczik2009a, abstract = {In their natural environment, many insects need to identify and evaluate behaviorally relevant odorants on a rich and dynamic olfactory background. Behavioral studies have demonstrated that bees recognize learned odors within {\textless}200 ms, indicating a rapid processing of olfactory input in the sensory pathway. We studied the role of the honeybee antennal lobe network in constructing a fast and reliable code of odor identity using in vivo intracellular recordings of individual projection neurons (PNs) and local interneurons (LNs). We found a complementary ensemble code where odor identity is encoded in the spatio-temporal pattern of response latencies as well as in the pattern of activated and inactivated PN firing. This coding scheme rapidly reaches a stable representation within 50-150 ms after stimulus onset. Testing an odor mixture versus its individual compounds revealed different representations in the two morphologically distinct types of lateral- and median PNs (l- and m-PNs). Individual m-PNs mixture responses were dominated by the most effective compound (elemental representation) whereas l-PNs showed suppressed responses to the mixture but not to its individual compounds (synthetic representation). The onset of inhibition in the membrane potential of l-PNs coincided with the responses of putative inhibitory interneurons that responded significantly faster than PNs. Taken together, our results suggest that processing within the LN network of the AL is an essential component of constructing the antennal lobe population code. {\textcopyright} 2009 Krofczik, Menzel and Nawrot.}, author = {Rybak, J{\"{u}}rgen and Ku{\ss}, Anja and Holler, Wolfgang and Brandt, Robert and Hege, Hans-Christian and Nawrot, Martin Paul and Menzel, Randolf}, booktitle = {BMC Neuroscience}, doi = {10.3389/neuro.10.009.2008}, file = {:Users/springer/Downloads/1471-2202-10-S1-P1.pdf:pdf}, issn = {16625188}, keywords = {Antennal lobe,Apis mellifera,Latency code,Local interneurons,Odor mixture,Olfaction,Projection neurons,Temporal coding}, number = {S1}, pages = {P1}, pmid = {19221584}, title = {{The HoneyBee Standard Brain (HSB) – a versatile atlas tool for integrating data and data exchange in the neuroscience community}}, url = {doi:10.1186/1471-2202-10-S1-P1}, volume = {10}, year = {2009} }
-
Farkhooi, F., Muller, E., & Nawrot, M. P.. (2009). Sequential sparsing by successive adapting neural populations. Paper presented at the BMC Neuroscience.
[Bibtex]@inproceedings{Farkhooi2009a, abstract = {In the principal cells of the insect mushroom body, the Kenyon cells (KC), olfactory information is represented by a spatially and temporally sparse code. Each odor stimulus will activate only a small portion of neurons and each stimulus leads to only a short phasic response following stimulus onset irrespective of the actual duration of a constant stimulus. The mechanisms responsible for the sparse code in the KCs are yet unresolved. Here, we explore the role of the neuron-intrinsic mechanism of spike-frequency adaptation (SFA) in producing temporally sparse responses to sensory stimulation in higher processing stages. Our single neuron model is defined through a conductance-based integrate-and-fire neuron with spike-frequency adaptation [1]. We study a fully connected feed-forward network architecture in coarse analogy to the insect olfactory pathway. A first layer of ten neurons represents the projection neurons (PNs) of the antenna lobe. All PNs receive a step-like input from the olfactory receptor neurons, which was realized by independent Poisson processes. The second layer represents 100 KCs which converge onto ten neurons in the output layer which represents the population of mushroom body extrinsic neurons (ENs). Our simulation result matches with the experimental observations. In particular, intracellular recordings of PNs show a clear phasic-tonic response that outlasts the stimulus [2] while extracellular recordings from KCs in the locust express sharp transient responses [3]. We conclude that the neuron-intrinsic mechanism is can explain a progressive temporal response sparsening in the insect olfactory system. Further experimental work is needed to test this hypothesis empirically. [1] Muller et. al., Neural Comput, 19(11):2958-3010, 2007. [2] Assisi et. al., Nat Neurosci, 10(9):1176-1184, 2007. [3] Krofczik et. al. Front. Comput. Neurosci., 2(9), 2009.}, author = {Farkhooi, Farzad and Muller, Eilif and Nawrot, Martin P}, booktitle = {BMC Neuroscience}, doi = {10.1186/1471-2202-10-s1-o10}, file = {:Users/springer/Downloads/1471-2202-10-S1-O10.pdf:pdf}, issn = {1471-2202}, number = {S1}, pages = {O10}, title = {{Sequential sparsing by successive adapting neural populations}}, url = {doi:10.1186/1471-2202-10-S1-O10}, volume = {10}, year = {2009} }
-
Nawrot, M. P., Aertsen, A., & Rotter, S.. (2003). Elimination of response latency variability in neuronal spike trains. Biological Cybernetics, 88(5), 321–334.
[Bibtex]@article{Nawrot2003, abstract = {Neuronal activity in the mammalian cortex exhibits a considerable amount of trial-by-trial variability. This may be reflected by the magnitude of the activity as well as by the response latency with respect to an external event, such as the onset of a sensory stimulus, or a behavioral event. Here we present a novel nonparametric method for estimating trial-by-trial differences in response latency from neuronal spike trains. The method makes use of the dynamic rate profile for each single trial and maximizes their total pairwise correlation by appropriately shifting all trials in time. The result is a new alignment of trials that largely eliminates the variability in response latency and provides a new internal trigger that is independent of experiment time. To calibrate the method, we simulated spike trains based on stochastic point processes using a parametric model for phasic response profiles. We illustrate the method by an application to simultaneous recordings from a pair of neurons in the motor cortex of a behaving monkey. It is demonstrated how the method can be used to study the temporal relation of the neuronal response to the experiment, to investigate whether neurons share the same dynamics, and to improve spike correlation analysis. Differences between this and other, previously published methods are discussed.}, author = {Nawrot, Martin P. and Aertsen, Ad and Rotter, Stefan}, doi = {10.1007/s00422-002-0391-5}, file = {::}, issn = {03401200}, journal = {Biological Cybernetics}, keywords = {method paper,variability}, mendeley-tags = {method paper,variability}, number = {5}, pages = {321--334}, title = {{Elimination of response latency variability in neuronal spike trains}}, volume = {88}, year = {2003} }
-
Egert, U., Knott, T., Schwarz, C., Nawrot, M. P., Brandt, A., Rotter, S., & Diesmann, M.. (2002). MEA-Tools: An open source toolbox for the analysis of multi-electrode data with MATLAB. Journal of Neuroscience Methods, 117(1), 33–42.
[Bibtex]@article{Egert2002, abstract = {Recent advances in electrophysiological techniques have created new tools for the acquisition and storage of neuronal activity recorded simultaneously with numerous electrodes. These techniques support the analysis of the function as well as the structure of individual electrogenic cells in the context of surrounding neuronal or cardiac network. Commercially available tools for the analysis of such data, however, cannot be easily adapted to newly emerging requirements for data analysis and visualization, and cross compatibility between them is limited. In this report we introduce a free open source toolbox called microelectrode array tools (MEA-Tools) for the analysis of multi-electrode data based on the common data analysis environment MATLAB (version 5.3-6.1, The Mathworks, Natick, MA). The toolbox itself is platform independent. The file interface currently supports files recorded with MCRack (Multi Channel Systems, Reutlingen, Germany) under Microsoft Windows 95, 98, NT, and 2000, but can be adapted to other data acquisition systems. Functions are controlled via command line input and graphical user interfaces, and support common requirements for the analysis of local field potentials, extracellular spike activity, and continuous recordings, in addition to supplementary data acquired by additional instruments, e.g. intracellular amplifiers. Data may be processed as continuous recordings or time windows triggered to some event. {\textcopyright} 2002 Elsevier Science B.V. All rights reserved.}, author = {Egert, U. and Knott, Th. and Schwarz, C. and Nawrot, M. P. and Brandt, A. and Rotter, S. and Diesmann, M.}, doi = {10.1016/S0165-0270(02)00045-6}, file = {::}, issn = {01650270}, journal = {Journal of Neuroscience Methods}, keywords = {Data analysis,Electrophysiology,Field potential analysis,Microelectrode arrays,Multi-electrode recording,Spike analysis}, number = {1}, pages = {33--42}, title = {{MEA-Tools: An open source toolbox for the analysis of multi-electrode data with MATLAB}}, volume = {117}, year = {2002} }
-
Nawrot, M. P., Aertsen, A., & Rotter, S.. (1999). Single-trial estimation of neuronal firing rates: From single-neuron spike trains to population activity. Journal of Neuroscience Methods, 94(1), 81–92.
[Bibtex]@article{Nawrot1999, abstract = {We present a method to estimate the neuronal firing rate from single-trial spike trains. The method, based on convolution of the spike train with a fixed kernel function, is calibrated by means of simulated spike trains for a representative selection of realistic dynamic rate functions. We derive rules for the optimized use and performance of the kernel method, specifically with respect to an effective choice of the shape and width of the kernel functions. An application of our technique to the on-line, single-trial reconstruction of arm movement trajectories from multiple single-unit spike trains using dynamic population vectors illustrates a possible use of the proposed method. Copyright (C) 1999 Elsevier Science B.V.}, author = {Nawrot, Martin P. and Aertsen, Ad and Rotter, Stefan}, doi = {10.1016/S0165-0270(99)00127-2}, file = {::}, issn = {01650270}, journal = {Journal of Neuroscience Methods}, keywords = {Dynamic population vector,Dynamic spike responses,Kernel estimator,Neural coding,Single-trial rate estimation,Spike train analysis,Stochastic point process,method paper}, mendeley-tags = {method paper}, number = {1}, pages = {81--92}, title = {{Single-trial estimation of neuronal firing rates: From single-neuron spike trains to population activity}}, volume = {94}, year = {1999} }