Anna-Maria Jürgensen
PhD Student
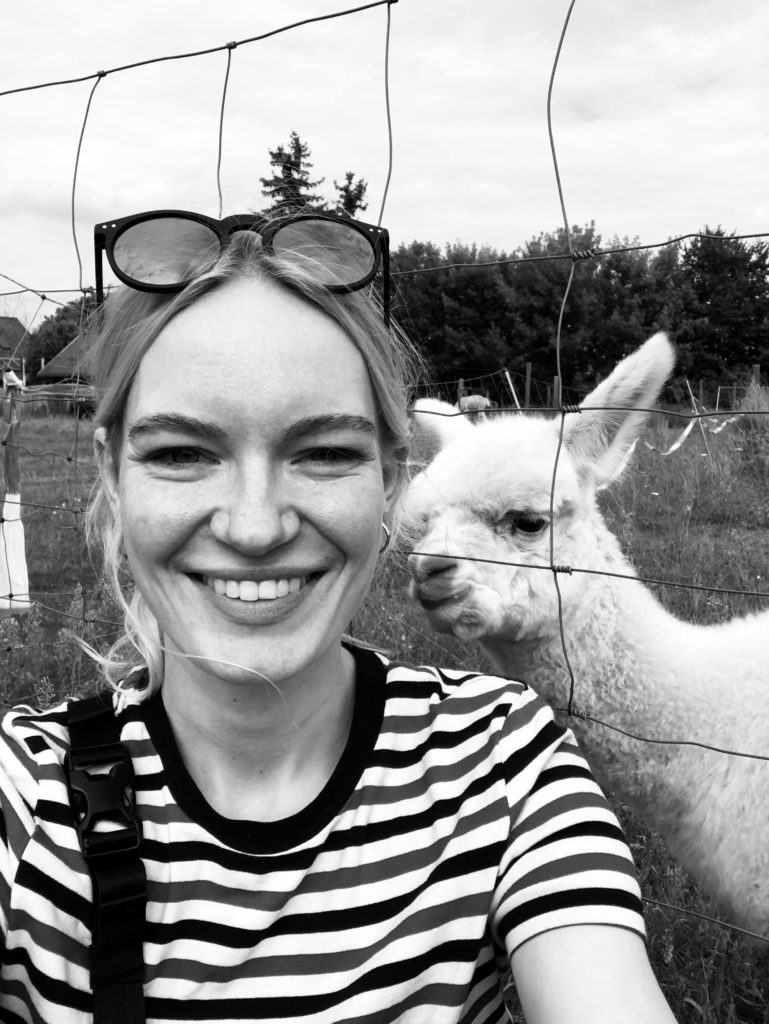
Room 1.318
Email: a.juergensen@uni-koeln.de
Phone: +49-221-470-76128
My work is centered around understanding the mechanisms underlying associative learning. I use computer simulations of rate and spiking network models of the Drosophila olfactory system to study learning through synaptic plasticity. In collaborations with experimental labs I focus on implementing the brain areas of interest with a high level of anatomical and functional accuracy to discover ‘how the brain actually does it’. I am especially interested in where and how prediction error signals are a biologically plausible driving force of plasticity in this circuit. Additionally I study how sparse code of higher-level sensory representations supports pattern discrimination and learning in the restricted coding space with its very limited number of neurons.
Publications (ORCID profile):
Articles
-
Sakagiannis, P., Jürgensen, A., & Nawrot, M. P.. (2025). A behavioral architecture for realistic simulations of Drosophila larva locomotion and foraging. eLife, 14, RP104262.
[Bibtex]@article{Sakagiannis_2025, title={A behavioral architecture for realistic simulations of Drosophila larva locomotion and foraging}, url={http://dx.doi.org/10.7554/eLife.104262.1}, DOI={10.7554/elife.104262.1}, publisher={eLife Sciences Publications, Ltd}, author={Sakagiannis, Panagiotis and Jürgensen, Anna-Maria and Nawrot, Martin Paul}, year={2025}, journal = {eLife}, volume = {14}, pages = {RP104262},month=apr }
-
Rachad, E. Y., Deimel, S. H., Epple, L., Gadgil, Y. V., Jürgensen, A., Springer, M., Lin, C., Nawrot, M. P., Lin, S., & Fiala, A.. (2025). Functional dissection of a neuronal brain circuit mediating higher-order associative learning. Cell Reports, 44(5), 115593.
[Bibtex]@article{RACHAD2025115593, title = {Functional dissection of a neuronal brain circuit mediating higher-order associative learning}, journal = {Cell Reports}, volume = {44}, number = {5}, pages = {115593}, year = {2025}, issn = {2211-1247}, doi = {https://doi.org/10.1016/j.celrep.2025.115593}, url = {https://www.sciencedirect.com/science/article/pii/S221112472500364X}, author = {Rachad, El Yazid and Deimel, Stephan Hubertus and Epple, Lisa and Gadgil, Yogesh Vasant and Jürgensen, Anna-Maria and Springer, Magdalena and Lin, Chen-Han and Nawrot, Martin Paul and Lin, Suewei and Fiala, André}, keywords = {associative learning, higher-order conditioning, mushroom body, , neuronal circuit dissection, learning and memory, insect brain, odor coding, dopamine, prediction error}, abstract = {A central feature characterizing the neural architecture of many species’ brains is their capacity to form associative chains through learning. In elementary forms of associative learning, stimuli coinciding with reward or punishment become attractive or repulsive. Notably, stimuli previously learned as attractive or repulsive can themselves serve as reinforcers, establishing a cascading effect whereby they become associated with additional stimuli. When this iterative process is perpetuated, it results in higher-order associations. Here, we use odor conditioning in Drosophila and computational modeling to dissect the architecture of neuronal networks underlying higher-order associative learning. We show that the responsible circuit, situated in the mushroom bodies of the brain, is characterized by parallel processing of odor information and by recurrent excitatory and inhibitory feedback loops that empower odors to gain control over the dopaminergic valence-signaling system. Our findings establish a paradigmatic framework of a neuronal circuit diagram enabling the acquisition of associative chains.} }
-
Jürgensen, A., Schmitt, F. J., & Nawrot, M. P.. (2024). Minimal circuit motifs for second-order conditioning in the insect mushroom body. Frontiers in Physiology, 14, 1326307.
[Bibtex]@article{10.3389/fphys.2023.1326307, abstract = {In well-established first-order conditioning experiments, the concurrence of a sensory cue with reinforcement forms an association, allowing the cue to predict future reinforcement. In the insect mushroom body, a brain region central to learning and memory, such associations are encoded in the synapses between its intrinsic and output neurons. This process is mediated by the activity of dopaminergic neurons that encode reinforcement signals. In second-order conditioning, a new sensory cue is paired with an already established one that presumably activates dopaminergic neurons due to its predictive power of the reinforcement. We explored minimal circuit motifs in the mushroom body for their ability to support second-order conditioning using mechanistic models. We found that dopaminergic neurons can either be activated directly by the mushroom body's intrinsic neurons or via feedback from the output neurons via several pathways. We demonstrated that the circuit motifs differ in their computational efficiency and robustness. Beyond previous research, we suggest an additional motif that relies on feedforward input of the mushroom body intrinsic neurons to dopaminergic neurons as a promising candidate for experimental evaluation. It differentiates well between trained and novel stimuli, demonstrating robust performance across a range of model parameters.}, author = {J{\"{u}}rgensen, Anna-Maria and Schmitt, Felix Johannes and Nawrot, Martin Paul}, doi = {10.3389/fphys.2023.1326307}, file = {:Users/springer/Downloads/fphys-14-1326307.pdf:pdf}, issn = {1664-042X}, journal = {Frontiers in Physiology}, month = {jan}, pages = {1326307}, title = {{Minimal circuit motifs for second-order conditioning in the insect mushroom body}}, url = {https://www.frontiersin.org/articles/10.3389/fphys.2023.1326307}, volume = {14}, year = {2024} }
-
Jürgensen, A., Sakagiannis, P., Schleyer, M., Gerber, B., & Nawrot, M. P.. (2024). Prediction error drives associative learning and conditioned behavior in a spiking model of Drosophila larva. iScience, 27(1).
[Bibtex]@article{Jurgensen2024, abstract = {Predicting reinforcement from sensory cues is beneficial for goal-directed behavior. In insect brains, underlying associations between cues and reinforcement, encoded by dopaminergic neurons, are formed in the mushroom body. We propose a spiking model of the Drosophila larva mushroom body. It includes a feedback motif conveying learned reinforcement expectation to dopaminergic neurons, which can compute prediction error as the difference between expected and present reinforcement. We demonstrate that this can serve as a driving force in learning. When combined with synaptic homeostasis, our model accounts for theoretically derived features of acquisition and loss of associations that depend on the intensity of the reinforcement and its temporal proximity to the cue. From modeling olfactory learning over the time course of behavioral experiments and simulating the locomotion of individual larvae toward or away from odor sources in a virtual environment, we conclude that learning driven by prediction errors can explain larval behavior.}, annote = {doi: 10.1016/j.isci.2023.108640}, author = {J{\"{u}}rgensen, Anna-Maria and Sakagiannis, Panagiotis and Schleyer, Michael and Gerber, Bertram and Nawrot, Martin Paul}, doi = {10.1016/j.isci.2023.108640}, issn = {2589-0042}, journal = {iScience}, month = {jan}, number = {1}, publisher = {Elsevier}, title = {{Prediction error drives associative learning and conditioned behavior in a spiking model of Drosophila larva}}, url = {https://doi.org/10.1016/j.isci.2023.108640}, volume = {27}, year = {2024} }
-
Jürgensen, A., Khalili, A., Chicca, E., Indiveri, G., & Nawrot, M. P.. (2021). A neuromorphic model of olfactory processing and sparse coding in the Drosophila larva brain. Neuromorphic Computing and Engineering.
[Bibtex]@article{Anna-MariaJurgensen*aAfshinKhalili*abElisabettaChiccacGiacomoIndiverid, author = {J{\"{u}}rgensen, Anna-Maria and Khalili, Afshin and Chicca, Elisabetta and Indiveri, Giacomo and Nawrot, Martin Paul}, doi = {10.1088/2634-4386/ac3ba6}, file = {:Users/springer/Downloads/J{\"{u}}rgensen+et+al{\_}2021{\_}Neuromorph.{\_}Comput.{\_}Eng.{\_}10.1088{\_}2634-4386{\_}ac3ba6.pdf:pdf}, isbn = {9781509008964}, issn = {13616579}, journal = {Neuromorphic Computing and Engineering}, month = {nov}, title = {{A neuromorphic model of olfactory processing and sparse coding in the Drosophila larva brain}}, url = {https://doi.org/10.1088/2634-4386/ac3ba6}, year = {2021} }
Preprints